GenAI: The Technology Shaping the Future
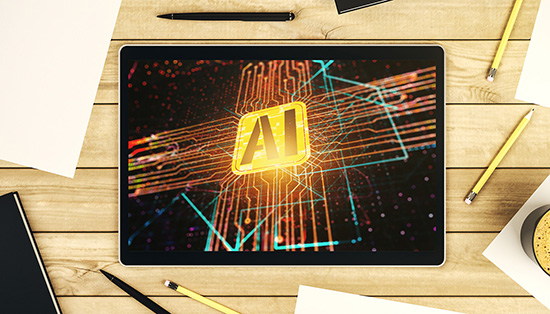
What is Generative Artificial Intelligence (GenAI)?
GenAI is a type of artificial intelligence capable of producing new and original content based on different data types such as text, images, audio, and video. This technology learns patterns and relationships within large datasets by analyzing them. For example, it can write a poem, draw a picture, or compose a piece of music. GenAI has the ability to create entirely new content that resembles or even surpasses human-made works.
Although its origins date back to the 1960s, it gained significant momentum in 2014 with the introduction of Generative Adversarial Networks (GANs). GANs involve two artificial intelligence models competing with each other to produce more realistic and high-quality content. One model generates content while the other evaluates whether the content is real, allowing both models to continuously improve.
GenAI can produce much deeper and more meaningful content by leveraging large language models (LLMs) and transformers. These models are trained on billions of data points found on the internet, enhancing their capacity to understand and generate human language, visuals, and other media types.
Thanks to new technologies like transformers, models can track connections between words not just within individual sentences but across pages, chapters, and books. This enables the creation of more complex and meaningful content.
How Does Generative Artificial Intelligence Work?
Fundamentals of Large Language Models
LLMs are particularly developed using Transformer architecture. Transformer architecture excels in understanding context within the text due to its attention mechanisms. This architecture, introduced by Vaswani et al. in 2017, has pioneered many innovations in the field of Natural Language Processing (NLP).
Components of Transformer Architecture
Input Representation: Text data is converted into numerical data that the model can understand. During this process, word embedding techniques are used to represent each word as a vector.
Self-Attention: The model attempts to understand the context by considering each word’s relationship with other words. It helps the model understand where the word is located in the sentence and how it interacts with other words.
Layers: A transformer consists of multiple layers, with each layer processing the information from the previous layer to better understand the context within the text.
Output Layer: After analyzing the text, the model produces the necessary output for a specific task. For example, tasks like text completion, question-answering, and translation are performed at this stage.
Training Process
LLMs are trained on vast amounts of text data. During the training process, the model learns the relationships between words and grasps the structure of the language. The data used in training are collected from various sources like books, articles, and websites. This extensive data enables the model to have a broad understanding of the language.
Applications of Large Language Models
Text Generation: LLMs can produce meaningful and coherent texts on a specific topic. They are used in content creation, software documentation, and creative writing.
Translation: LLMs are quite successful in translating texts between languages. Applications like Google Translate use this technology.
Question-Answering: LLMs can understand user questions and provide accurate answers. They are common in customer service and knowledge base systems.
Text Analysis: LLMs perform tasks such as sentiment analysis, summarization, and topic detection by analyzing text data.
Challenges and Future Perspectives
The development and use of LLMs bring certain challenges. These challenges include the need for large datasets, intensive use of computational resources, and ethical considerations. However, the development of LLMs continues rapidly, and efforts are ongoing to make these models more efficient, reliable, and ethical.
Advantages, Use Cases, and Future of Generative Artificial Intelligence
GenAI is still an evolving technology. However, it is expected to play a significant role in many different areas in the near future. Here are some potential use cases:
Software Development: Generative AI provides a 30-50% increase in efficiency in software development processes. This significantly reduces the working time of developers through automated processes like code completion and error correction. (BCG Global)
Customer Service: Generative AI-based virtual assistants improve customer service by up to 50%. This technology halves the duration of customer service calls in call centers. (BCG Global)
Cybersecurity: Generative AI can be used to detect and prevent cybersecurity threats. This significantly reduces the response time to security incidents and allows companies to close security gaps more quickly. (NVIDIA Blog)
Enhancing Creativity: It supports human creativity by generating new ideas and developing existing concepts. Fashion design companies can create new collections 30% faster using Generative AI (McKinsey & Company) (Nutanix)
Increasing Efficiency: It saves time and money by automating repetitive content creation tasks. Content marketing agencies can reduce content production time by 40% with Generative AI (Nutanix)
Increasing Personalization: It can create personalized product recommendations, marketing, and advertising, as well as educational and training materials for users. E-commerce sites can increase sales by 20% through personalized recommendations (McKinsey & Company)
Improving Products and Services: It helps develop new products and retail experiences. Retail stores can improve customer experience by 25%. (B2B Business Directory)
Advancing Scientific Research: It can be used to analyze and model complex datasets, form new hypotheses and theories, and design and conduct scientific experiments. Genetic research can perform data analysis 35% faster. (McKinsey & Company)
Social Impact: It develops assistive technologies for the disabled and tools for emergency management. It can be used to manage natural disasters and emergencies and improve education and training. It can increase prediction accuracy in natural disaster management by 40%. (quantzig)
Ethical Dimensions of Generative Artificial Intelligence
While GenAI has many benefits, some ethical dimensions cannot be ignored. Some ethical issues to consider are:
Misinformation and Disinformation: It can be used to produce false information and propaganda.
Bias and Discrimination: It can reflect biases in training data, leading to discriminatory outcomes.
Ownership and Copyright: There are uncertainties about the ownership and copyright of the generated content.
It is important to pay attention to these ethical dimensions and to develop and use GenAI responsibly.
Source: What is Generative AI? Everything You Need to Know (techtarget.com) and GPT-4o
Author: Mert Badalıoğlu, GTech Big Data & Analytics Consultant